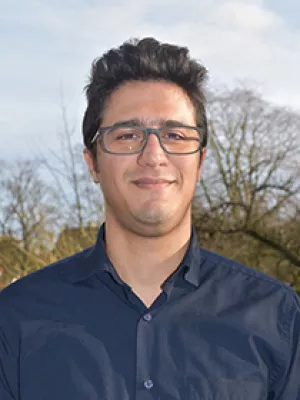
Amir Naghibi
Researcher
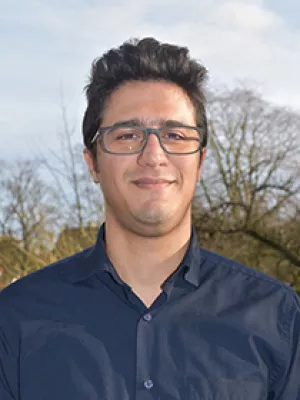
Robust probabilistic modelling of mould growth in building envelopes using random forests machine learning algorithm
Author
Summary, in English
Probabilistic methods can be used to account for uncertainties in hygrothermal analysis of building envelopes. This paper presents methods for robust mould reliability analysis and identification of critical parameters. Mould indices are calculated by probabilistic hygrothermal analysis, followed by the application of the "Finnish mould growth model." To increase the robustness of the mould growth analysis, a random forests metamodel is first trained on the dataset and then used to expand the number of simulations. Finally, the reliability is calculated based on the probability of exceeding a given maximum mould index limit state. Critical parameters are identified through a sensitivity analysis based on linear and non-linear dependencies between inputs and maximum mould index. The methods are demonstrated by analysing three external wall assemblies. In conclusion, the mould reliability analysis method helps to assess the robustness of the hygrothermal analysis and mould assessment by investigating the influence of hygrothermal variables' uncertainties on the maximum mould index. By combining a metamodel with probabilistic analysis, it is possible to significantly reduce the amount of time required to evaluate a large number of scenarios.
Department/s
- Division of Structural Engineering
- Division of Water Resources Engineering
- LTH Profile Area: Water
- Centre for Advanced Middle Eastern Studies (CMES)
- MECW: The Middle East in the Contemporary World
Publishing year
2023
Language
English
Publication/Series
Building and Environment
Volume
243
Document type
Journal article
Publisher
Elsevier
Topic
- Building Technologies
Keywords
- Building envelope
- Hygrothermal simulation
- Machine learning
- Mould assessment
- Reliability analysis
- Sensitivity analysis
Status
Published
ISBN/ISSN/Other
- ISSN: 0360-1323