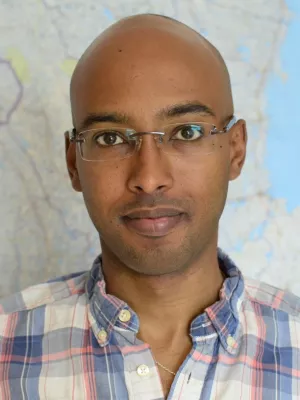
Hakim Abdi
Researcher
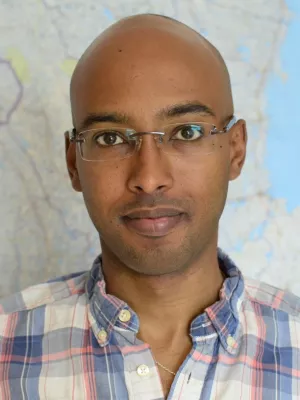
Urban land use and land cover classification with interpretable machine learning – A case study using Sentinel-2 and auxiliary data
Author
Summary, in English
The European commission launch of the twin Sentinel-2 satellites provides new opportunities for land use and land cover (LULC) classification because of the readily availability of their data and their enhanced spatial, temporal and spectral resolutions. The rapid development of machine learning over the past decade led to data-driven models being at the forefront of high accuracy predictions of the physical world. However, the contribution of the driving variables behind these predictions cannot be explained beyond generalized metrics of overall performance. Here, we compared the performance of three shallow learners (support vector machines, random forest, and extreme gradient boosting) as well as two deep learners (a convolutional neural network and a residual network with 50 layers) in and around the city of Malmö in southern Sweden. Our complete analysis suite involved 141 input features, 85 scenarios, and 8 LULC classes. We explored the interpretability of the five learners using Shapley additive explanations to better understand feature importance at the level of individual LULC classes. The purpose of class-level feature importance was to identify the most parsimonious combination of features that could reasonably map a particular class and enhance overall map accuracy. We showed that not only do overall accuracies increase from shallow (mean = 84.64%) to deep learners (mean = 92.63%) but that the number of explanatory variables required to obtain maximum accuracy decreases along the same gradient. Furthermore, we demonstrated that class-level importance metrics can be successfully identified using Shapley additive explanations in both shallow and deep learners, which allows for a more detailed understanding of variable importance. We show that for certain LULC classes there is a convergence of variable importance across all the algorithms, which helps explain model predictions and aid the selection of more parsimonious models. The use of class-level feature importance metrics is still new in LULC classification, and this study provides important insight into the potential of more nuanced importance metrics.
Department/s
- Transport and Roads
- Centre for Environmental and Climate Science (CEC)
- BECC: Biodiversity and Ecosystem services in a Changing Climate
Publishing year
2022-09-29
Language
English
Publication/Series
Remote Sensing Applications: Society and Environment
Volume
28
Document type
Journal article
Publisher
Elsevier
Topic
- Remote Sensing
- Physical Geography
- Environmental Sciences
Keywords
- Remote sensing
- Machine learning
- Deep learning
- Explainable AI
- Earth observation
- Sweden
Status
Published
ISBN/ISSN/Other
- ISSN: 2352-9385